Assistant Professor, Department of Biomedical Informatics
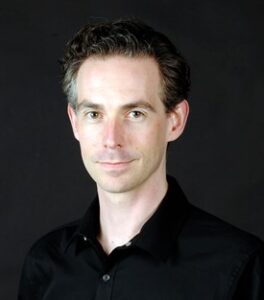
Title: Quantitative Imaging Analysis and Genomics of Multiple Myeloma
Imaging data are routinely used in the diagnosis and treatment of many cancers, but they are mostly used qualitatively, not quantitatively. Multiple myeloma (MM) is a plasma cell cancer that resides in the bone marrow and results in over 30,000 new cases and over 12,000 deaths each year in the United States. The long-term goal of this project is fully quantitative analysis of MM imaging data and its integration with clinical, molecular, and genomic data. Imaging using positron emission tomography-computed tomography (PET/CT) is standard in MM diagnosis and treatment, as it allows detection and monitoring of two types of lesions. Focal lesions (FL) are agglomerations of cancer cells and represent sites of intensely active disease, which are visible in PET. Frequently associated with FL are osteolytic bone lesions (OL), regions of extremely low bone density which lead to bone pain and fractures which can be catastrophically debilitating and are visible in CT. Dr. Wardell’s hypothesis is that rapid, reproducible, and quantitative image analysis will lead directly to an improved understanding of the disease physiology of MM patients and these new insights will quickly lead to improved clinical outcomes. He will use state-of-the-art machine learning methods to produce whole-skeleton segmentations from any whole-body CT scan and use current clinical standards to identify both FL and OL. The main objective of this proposal is to develop automated methods for segmenting bones and myeloma lesions in PET/CT scans and to integrate these quantitative imaging data with molecular and clinical data. Segmenting bones from CT scans is a crucial missing piece of technology on which further studies depend. An automated machine-learning approach will allow users to perform faster and at scales impossible when using laborious, time-consuming manual segmentation. Aim 1 will train a machine learning model for whole-skeleton bone segmentation from CT data. Aim 2 will develop methods and software for detection, measurement, annotation, and visualization of myeloma lesions both in PET and CT scans. Aim 3 will integrate multiple myeloma imaging data with clinical and molecular data.