Dr. Melody Greer, an assistant professor in the Department of Biomedical Informatics (DBMI), has published several articles in recent months, including topics in machine learning (ML), artificial intelligence (AI) and potential bias in algorithms.
“Repeatable enhancement of healthcare data with social determinants of health” was published in Frontiers in Big Data in August 2022 by Dr. Greer, first author, along with DBMI graduate student Cilia Zayas and Sudeepa Bhattacharyya of Arkansas State University and UAMS.

The research team developed a repeatable social determinants of health (SDOH) enrichment and integration process to incorporate dynamically evolving SDOH domain concepts from consumer data into clinical data. This process included SDOH mapping, linking compiled consumer data to patient records in electronic health records (EHR), data quality analysis and preprocessing, and storage. Their analysis showed commercial consumer data can be a viable source of SDOH data at an individual level, providing a path for clinicians to improve patient treatment and care. This enhanced data will support machine learning models for early prediction of colorectal cancer risk, prognosis, and survival.

“Machine learning can identify patients at risk of hyperparathyroidism without known calcium and intact parathyroid hormone” was published in Head & Neck in April 2022, with Dr. Greer as first author, along with co-authors Drs. Kyle Davis and Brendan C Stack Jr., both formerly of the UAMS Department of Otolaryngology.
The authors did a proof-of-concept investigation for diagnosing primary hyperparathyroidism under certain permeators. Using a dataset from the UAMS Epic electronic health record system, they were able to predict primary hyperparathyroidism with 86% accuracy. The approach will now be validated and refined, and plans are in place to attempt it with other regional/national datasets.
“Preprocessing to address bias in healthcare data” was published in Studies in Health Technology and Informatics in May 2022, with DBMI data analyst Emel Seker as first author and Dr. Greer as senior author, with assistance from John Talburt of the University of Arkansas at Little Rock.
The group hypothesized that people living in rural settings are less likely to have the full list of their multiple chronic health conditions included in the electronic health record. Therefore, their data may not be accurately informing artificial intelligence (AI) algorithms. This situation could create bias against people living in rural areas of AI tools are trained on data from metropolitan areas. The team created a model to test their hypothesis, and found evidence to suggest there is indeed bias against rural patients for algorithms that rely on diagnostic information to calculate risk.
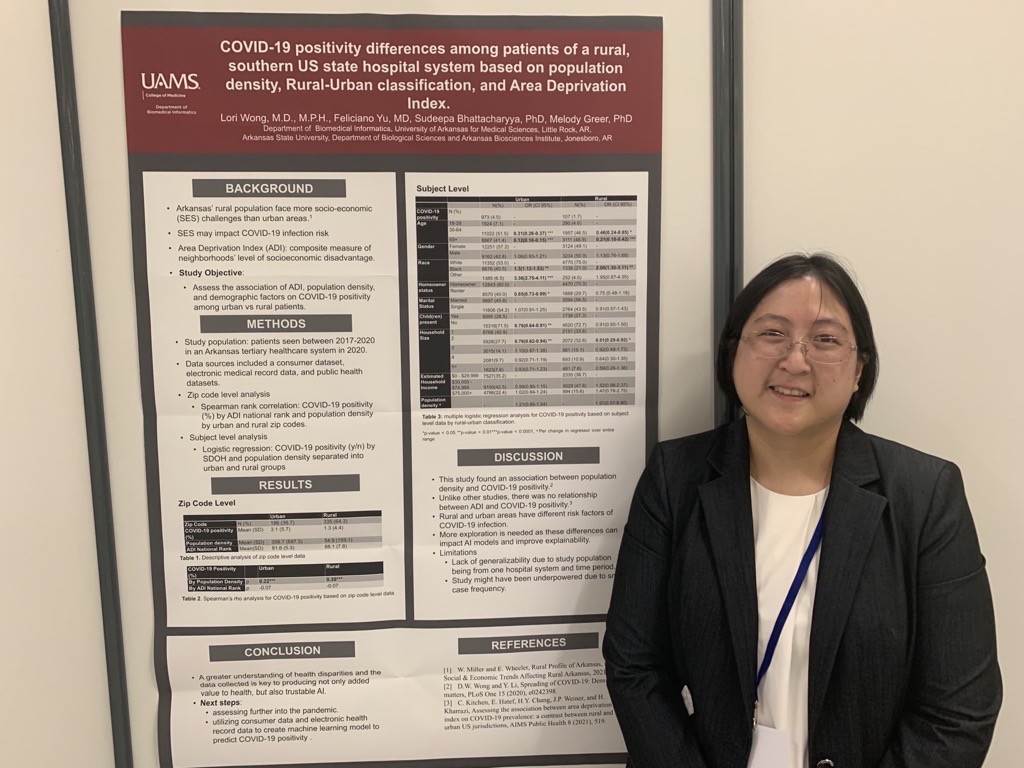
Dr. Greer was also the senior author on a paper by Clinical Informatics Fellow Dr. Lori Wong, “COVID-19 positivity differences among patients of a rural, southern US state hospital system based on population density, rural-urban classification, and area deprivation index” was published in Studies in Health Technology and Informatics in May 2022. Co-authors include Dr. Pele Yu with DBMI and Sudeepa Bhattacharyya of Arkansas State University and UAMS.
They examined the correlation of COVID-19 positivity with indexes meant to measure various social determinants of health. They found that COVID-19 positivity was significantly associated with population density, age, race, and household size. The researchers aimed to better understand how health disparities data can add value to health and the creation of trustable AI.